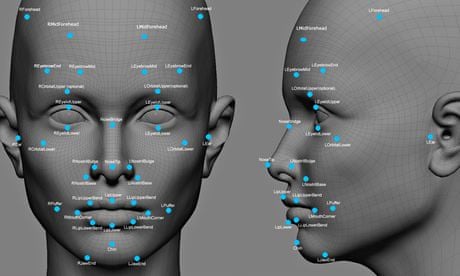
Facial Recognition Software, Race, Gender Bias, and Policing
April 11, 2020Archives . Authors . Blog News . Certified Review . Feature . Feature Img . Issue Spotters . Notes . Policy/Contributor Blogs . Recent Stories . Student Blogs Article(Source)
Facial Recognition Technology (FRT) identifies a person’s face by navigating through computer programs that access thousands of cameras worldwide to identify a suspect, criminal or fugitive. FRT could even accurately identify a person’s from a blurry captured image or instantaneously identify the subject among a crowd. This is the fantasy portrayed in Hollywood movies. In reality, facial recognition software is inaccurate, bias, and under-regulated.
FRT creates a facial template of a person’s facial image and compares the template to millions of photographs stored in databases—driver’s license, mugshots, government records, or social media accounts. While this technology aims to accelerate law enforcement investigative work and more accurately identify crime suspects, it has been criticized for its bias against African-American Women. The arm of the United States government responsible for establishing standards for technology—National Institute For Standards and Technology (NIST)—conducted a test in July of 2019 that showed FRT’s bias against African-American women. NIST tested Idemia’s algorithms—an Australian company that provides facial recognition software to police in United States—for accuracy. The software mismatched white women’s faces once in 10,000, but it mismatched black women’s faces once in 1000 instances.
This disparity is a result of the failure of current facial algorithms to recognize darker skin and is not limited to Idemia’s software. In fact, NIST reported similar results from 50 different companies. Moreover, facial recognition software that relies on mugshots to identify suspects captured on camera or video likely includes a disproportionate number of African-American due to higher arrest rates. This inaccuracy could lead to false arrest. For instance, police used facial recognition software in Fergusson, Missouri to arrest protesters following the death of Michael Brown. Considering the high mismatch rate for African-Americans, police could have arrested peaceful protesters due to false positives. Another prominent instance of facial recognition software’s mismatch is Amazon’s Rekognition software—a software that uses learning technology to identify objects and peoples’ faces . The software disproportionately mismatched 28 members of congress. One mismatch was civil rights activist Rep. John Lewis of Georgia.
Facial recognition software could arguable affect police conduct investigations. For instance, facial recognition software failed to identify the Boston Marathon terrorists—Tsarnaev brothers—with pale skin. But it could have falsely identified an African-American in police database, assuming the suspect is black. Infact, a Massachusetts Institute of Technology research described positive identification of African-American women as a “coin toss.” While NIST publishes its findings on facial recognition software, little to no law exists to regulate the use of this technology.
No state in the United States has a comprehensive law regulating law enforcement’s use of facial recognition software. Without laws limiting the use of this software, it is prone to misuse. Neither search warrant nor reasonable suspicion is required to use FRT in identifying suspects. Law enforcement agencies may be pressured to make an arrest when FRT identifies a person as fitting a suspect. For instance in the Boston Marathon bombing, it was critical to quickly identify and arrest the suspect before he struck again. Granted, FRT has been useful in a number of situations. For instance, the Los Angeles Police Department quickly apprehended a dangerous suspect wanted in a fatal shooting case using FRT. But law enforcement agencies are not transparent with the use of this technology. A Georgetown Law Center on Privacy and Technology report finds that only 4 out of 52 agencies surveyed have a publicly available use policy. Maryland’s facial recognition software has never been audited for misuse. Even if suspects have been misidentified, there is no way for the public to know because no record exists.
Comprehensive laws guiding the use of FRT ought to be enacted on the federal level as a counterpart to the Wiretap Act. Although FRT is still developing, Congress ought to enact laws to guide its use in the right direction, especially in areas of racial and gender bias.
About the Author: Oluwasegun Joseph is a Third-year Cornell Law Student who enjoys following developing stories at the intersection of public policy and law. One of his goals is to become a federal prosecutor.
Suggested Citation: Oluwasegun Joseph, Facial Recognition Software, Race, Gender Bias, and Policing, Cornell J.L. & Pub. Pol’y, The Issue Spotter, (Apr. 10, 2020), https://live-journal-of-law-and-public-policy.pantheonsite.io/facial-recognition-software-race-gender-bias-and-policing.
You may also like
- November 2024
- October 2024
- April 2024
- March 2024
- February 2024
- November 2023
- October 2023
- April 2023
- March 2023
- February 2023
- January 2023
- December 2022
- November 2022
- October 2022
- May 2022
- April 2022
- March 2022
- February 2022
- January 2022
- December 2021
- November 2021
- October 2021
- May 2021
- April 2021
- March 2021
- February 2021
- January 2021
- November 2020
- October 2020
- September 2020
- August 2020
- July 2020
- June 2020
- May 2020
- April 2020
- March 2020
- February 2020
- January 2020
- November 2019
- October 2019
- September 2019
- April 2019
- February 2019
- December 2018
- November 2018
- October 2018
- September 2018
- March 2018
- February 2018
- January 2018
- December 2017
- November 2017
- October 2017
- September 2017
- May 2017
- April 2017
- March 2017
- February 2017
- December 2016
- November 2016
- October 2016
- April 2016
- March 2016
- February 2016
- January 2016
- December 2015
- November 2015
- October 2015
- June 2015
- May 2015
- April 2015
- March 2015
- February 2015
- January 2015
- December 2014
- November 2014
- October 2014
- August 2014
- March 2014
- February 2014
- January 2014
- December 2013
- November 2013
- October 2013
- September 2013
- May 2013
- April 2013
- March 2013
- February 2013
- January 2013
- December 2012
- November 2012
- October 2012
- September 2012
- June 2012
- April 2012
- March 2012
- February 2012
- January 2012
- December 2011
- November 2011
- October 2011
- September 2011
- August 2011
- April 2011
- March 2011
- November 2010
- October 2010
- September 2010